Since ChatGPT was released just about a year ago, generative AI tools with chat interfaces have quickly permeated many aspects of our lives, including education. Additional large language models, such as Bard and Claude, have joined GPT as foundational to many applications. These are now being integrated with other tools for image creation, data visualization, tutoring, coding, knowledge management and much more. Most significantly for education, they are being incorporated into the word processing, presentation, and other productivity tools that teachers and students use every day. Everyone – including those who created the foundational generative AI systems – has been surprised by the capacities of the new AI tools. For example, Bill Gates reported that he was “in a state of shock“ when he first realized what these systems can do.
As educators, we are just beginning to explore the potential benefits and recognize the risks of students and teachers using generative AI tools. In doing so, we need to develop our understanding of the new AI, what it is and what it is not, since how we think about it will influence our decisions about its use. In this blog, I share a few thoughts about my developing understanding of the new AI.
First, generative AI is not simply the next generation in the line of technological advances that impact education, from calculators to internet search engines to mobile devices. Each generation raised both hopes for how they could enhance and fears for how they might harm students’ learning. Over time, educators developed ways to incorporate each technology productively, adjusting learning goals and activities and establishing appropriate use guidelines. If we adopt this “just the latest technology tool” view, the message to educators would be to pilot new AI tools, evaluate the results, and gradually incorporate AI into current practices, perhaps with some adjustments. This perspective could be limiting and work against updating the goals and practices of education to prepare students for their futures.
Generative AI changes the paradigm we all learned that computers require detailed, step-by-step instructions written in a programming language for each specific capability they provide. In this paradigm, any incorrect output is due to a “bug” in the program or inaccurate input data. These rule-based programmed systems, while yielding significant advances, never approached human abilities for things like language processing and picture recognition.
Generative AI systems are built with machine learning algorithms that extract patterns from enormous data sets. The extracted information is captured in digital neural networks that are somewhat modeled on the neurons and synapses of the human brain. That is, generative AI systems build a knowledge structure — the neural network — from the data on which they are trained, and then use that knowledge to generate novel responses to prompts. The result is that generative AI systems can respond to inputs in English and other languages, carry out dialogs that come close to meeting the Turing test of being indistinguishable from human interactions, and do many things well beyond the capabilities of prior systems, such as to create novel artistic pictures, solve long-standing scientific problems, navigate busy streets, and, of course, write all kinds of texts and even mimic the styles of specific human authors. Generative AI brings to school something very different, far more powerful, and more easily used than anything we had before.
Generative AI systems’ responses can seem very human-like. This can lead to the view that AI could replace humans, including teachers, which I consider a fallacious idea that would be damaging if applied in education.
When considering how generative AI should be used in education, it is essential to recognize that artificial and human intelligence are very different. A starting point to distinguish AI and human intelligence is to consider how each learns. AI learns from the data on which it is trained, while humans learn from a wide range of experiences in the world. Large Language Models can be said to have only book (i.e., text) knowledge in that all they “know” is derived from the enormous amount of text on which they are trained. Human learning is much richer, involving experience in the physical world, learning through play, motivation to accomplish goals, modeling of other people, and interactions within families, communities and cultures, as well as learning from texts and other media and formal learning in schools. Human babies have innate abilities to learn to perceive the world, develop social interactions, and master spoken languages. Human learning is ongoing throughout one’s life. It involves developing an understanding of causes and effects, human emotions, a sense of self, empathy for others, moral principles, the ability to understand the many subtleties of human interactions, and so much more that makes humans human. AI does match the richness of human experience, knowledge and intuition.
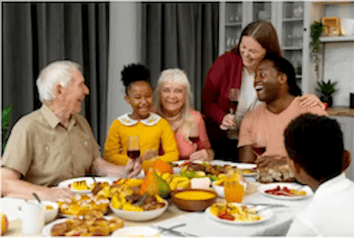
Human vs. AI understanding: A family holiday dinner.
As an example of how human and machine intelligence differ, contrast your thoughts and feelings when viewing the picture of a family holiday dinner with what an AI model could “know.” AI cannot know the power of the relationships and the meanings and emotions of people celebrating a tradition together. AI can identify the foods and provide recipes, but cannot experience the tastes, aromas and textures of the dishes, or the joy of preparing a delicious meal and sharing it with loved ones. While an AI can produce reasonable-sounding language about all those things, it does so by mimicking information it has absorbed without any human-like understanding, so it cannot come close to capturing what humans will see, know and feel about it. That is, AI cannot replicate or replace the human relationships and understandings that are essential for successful teaching and learning.
If generative AI is neither just another step in technology advances nor a replacement for human intelligence, how might we think about it? Another view is that AI can be considered a non-human, almost alien-like, intelligence that differs from humans but can augment our capabilities. Chris Dede frames this well with an analogy from Star Trek of the human Captain Picard and the artificially intelligent android Data (comparable to Captain Kirk and Mr. Spock in the earlier series). The human leader and the logical, data-driven assistant work together successfully navigating the many challenges they face while traversing the universe, accomplishing things that neither could achieve without the other. But in all cases, the human Captain Picard sets the goals and makes the critical decisions and android Data serves to help meet those goals. This pairing of human and non-human intelligence can serve as an analogy to the view that AI has many capabilities to help us meet educational goals, but always at the service of teachers and students.
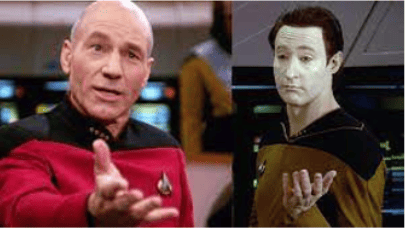
Captain Picard and Data: Different Types of Intelligence Working Together
This framing of “humans lead and AI serves“ is a productive one to inform planning to use generative AI in education. It leads to thinking about how AI agents can play many roles that help teachers and students–planning lessons, analyzing students’ progress, providing feedback, facilitating classroom interactions and collaborative work, enabling creative work, and so many more–including many we have not yet discovered. That is, AI agents will be our assistants and collaborators, bringing their unique but different skills to help us meet our educational goals.
Punya Mishra, in his blog on Teacher Knowledge in the Age of ChatGPT, eloquently captures the future of humans and AI working together:
GenAI doesn’t just operate in isolation, but it interacts, learns, and grows through dialogue with humans. This collaborative dance of information exchange collapses the old boundaries that once defined our relationship with tools and technology…. How we make sense of these new tools is emergent based on multiple rounds of dialogue and interactions with them…. Thus, we’re not just users or operators, we’re co-creators, shaping and being shaped by these technologies in a continuous and dynamic process of co-constitution.
Exciting times are ahead as we determine how to effectively partner with – not just employ – generative AI to enhance teaching and learning and address the many challenges of education.
Author: Glenn M. Kleiman, Senior Advisor, Stanford Accelerator for Learning, Stanford Graduate School of Education
Member of CoSN’s EdTech Innovations Committee
Published on: October 31 2023
CoSN is vendor neutral and does not endorse products or services. Any mention of a specific solution is for contextual purposes.